Gillespie Algorithm#
from random import choices, expovariate
import numpy as np
import matplotlib.pyplot as plt
%matplotlib inline
"""
Stochastic chemical reaction: Gillespie Algorithm
Adapted from: Chemical and Biomedical Enginnering Calculations Using Python Ch.4-3
Reaction of A <-> B with rate constants k1 & k2
"""
class Gillespie():
def __init__(self, propensityFuncs, actionFuncs, parameters=None):
self.propensityFuncs = propensityFuncs
self.actionFuncs = actionFuncs
self.parameters = parameters
def run(self, u0, tend, tstart=0):
# Setup
t = tstart
p = self.parameters
u = np.asarray(u0)
us = [u.copy()]
ts = [t]
while t < tend:
# propensities of reactions
ps = [f(u, p, t) for f in self.propensityFuncs]
pTotal = sum(ps)
dt = expovariate(pTotal)
# Choose an action by the weight of each propensities, and update the state variable
act = choices(actionFuncs, weights=ps)[0]
u = np.asarray(act(u, p, t))
t += dt
us.append(u.copy())
ts.append(t)
return np.array(ts), np.array(us)
parameters = {"k1": 1.0, "k2": 0.5}
propensityFuncs = (lambda u, p, t: p["k1"] * u[0],
lambda u, p, t: p["k2"] * u[1])
actionFuncs = (lambda u, p, t: [u[0] - 1, u[1] + 1],
lambda u, p, t: [u[0] + 1, u[1] - 1])
ssa = Gillespie(propensityFuncs = propensityFuncs,
actionFuncs = actionFuncs,
parameters = parameters)
ts, us = ssa.run([175, 25], 10.0)
fig, ax = plt.subplots()
ax.plot(ts, us[:, 0], label="A")
ax.plot(ts, us[:, 1], label="B")
ax.set_xlabel('time')
ax.set_ylabel('number of molecules')
ax.legend(loc='best')
<matplotlib.legend.Legend at 0x7f8b37531a90>
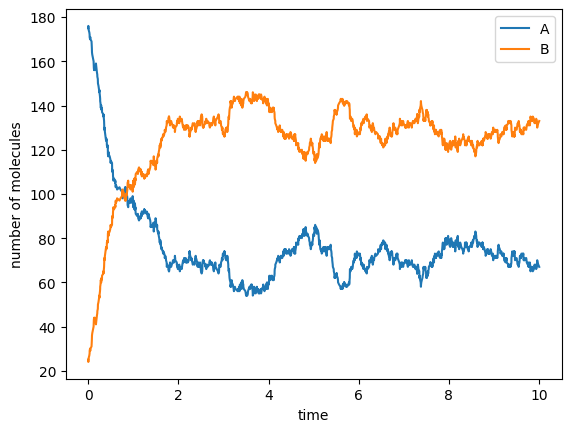