Using pandas#
import pandas as pd
import numpy as np # for generating random numbers
Series#
# series
s1 = pd.Series([10, 11, 12], dtype = float)
s1
0 10.0
1 11.0
2 12.0
dtype: float64
s2 = pd.Series([10, 11, 12], dtype = int)
s2
0 10
1 11
2 12
dtype: int64
s3 = pd.Series(["10", "11", "12"], name = 'Val', index = pd.Index(['a', 'b', 'c'], name = 'label'))
s3
label
a 10
b 11
c 12
Name: Val, dtype: object
s1.index
RangeIndex(start=0, stop=3, step=1)
# s1.append(s2)
s4 = pd.concat([s1, s3])
s4
0 10.0
1 11.0
2 12.0
a 10
b 11
c 12
dtype: object
print(s4[0])
print(s4)
s4_reset = s4.reset_index(drop=True)
print(s4_reset)
s4_reset[0]
10.0
0 10.0
1 11.0
2 12.0
a 10
b 11
c 12
dtype: object
0 10.0
1 11.0
2 12.0
3 10
4 11
5 12
dtype: object
10.0
dates = pd.date_range('20190503', periods=7)
dates
DatetimeIndex(['2019-05-03', '2019-05-04', '2019-05-05', '2019-05-06',
'2019-05-07', '2019-05-08', '2019-05-09'],
dtype='datetime64[ns]', freq='D')
DataFrame#
df = pd.DataFrame(np.random.randn(7, 4), index=dates, columns=list('ABCD'))
df
A | B | C | D | |
---|---|---|---|---|
2019-05-03 | -2.124415 | -0.024856 | 1.419856 | 0.345841 |
2019-05-04 | 1.181768 | -0.034609 | -1.540023 | -0.807267 |
2019-05-05 | -0.590696 | -2.111859 | -0.522006 | 1.315965 |
2019-05-06 | 0.441328 | -0.132428 | -0.397920 | -0.087632 |
2019-05-07 | -0.447503 | 0.303955 | -0.272940 | 0.169369 |
2019-05-08 | 0.298479 | -0.755295 | -0.728532 | 1.162973 |
2019-05-09 | 1.070966 | -0.004473 | 0.928714 | 1.486172 |
df2 = pd.DataFrame({'A': 1.,
'B': pd.Timestamp('20130102'),
'C': pd.Series(1, index=list(range(2, 6)), dtype='float32'),
'D': np.array([3] * 4, dtype='int32'),
'E': pd.Categorical(["test", "train", "test", "train"]),
'F': 'foo'})
df2
A | B | C | D | E | F | |
---|---|---|---|---|---|---|
2 | 1.0 | 2013-01-02 | 1.0 | 3 | test | foo |
3 | 1.0 | 2013-01-02 | 1.0 | 3 | train | foo |
4 | 1.0 | 2013-01-02 | 1.0 | 3 | test | foo |
5 | 1.0 | 2013-01-02 | 1.0 | 3 | train | foo |
df2.dtypes
A float64
B datetime64[s]
C float32
D int32
E category
F object
dtype: object
df = pd.read_csv('heart.csv')
df
Unnamed: 0 | Age | Sex | ChestPain | RestBP | Chol | Fbs | RestECG | MaxHR | ExAng | Oldpeak | Slope | Ca | Thal | AHD | |
---|---|---|---|---|---|---|---|---|---|---|---|---|---|---|---|
0 | 1 | 63 | 1 | typical | 145 | 233 | 1 | 2 | 150 | 0 | 2.3 | 3 | 0.0 | fixed | No |
1 | 2 | 67 | 1 | asymptomatic | 160 | 286 | 0 | 2 | 108 | 1 | 1.5 | 2 | 3.0 | normal | Yes |
2 | 3 | 67 | 1 | asymptomatic | 120 | 229 | 0 | 2 | 129 | 1 | 2.6 | 2 | 2.0 | reversable | Yes |
3 | 4 | 37 | 1 | nonanginal | 130 | 250 | 0 | 0 | 187 | 0 | 3.5 | 3 | 0.0 | normal | No |
4 | 5 | 41 | 0 | nontypical | 130 | 204 | 0 | 2 | 172 | 0 | 1.4 | 1 | 0.0 | normal | No |
... | ... | ... | ... | ... | ... | ... | ... | ... | ... | ... | ... | ... | ... | ... | ... |
298 | 299 | 45 | 1 | typical | 110 | 264 | 0 | 0 | 132 | 0 | 1.2 | 2 | 0.0 | reversable | Yes |
299 | 300 | 68 | 1 | asymptomatic | 144 | 193 | 1 | 0 | 141 | 0 | 3.4 | 2 | 2.0 | reversable | Yes |
300 | 301 | 57 | 1 | asymptomatic | 130 | 131 | 0 | 0 | 115 | 1 | 1.2 | 2 | 1.0 | reversable | Yes |
301 | 302 | 57 | 0 | nontypical | 130 | 236 | 0 | 2 | 174 | 0 | 0.0 | 2 | 1.0 | normal | Yes |
302 | 303 | 38 | 1 | nonanginal | 138 | 175 | 0 | 0 | 173 | 0 | 0.0 | 1 | NaN | normal | No |
303 rows × 15 columns
Viewing Data#
df.head(10)
Unnamed: 0 | Age | Sex | ChestPain | RestBP | Chol | Fbs | RestECG | MaxHR | ExAng | Oldpeak | Slope | Ca | Thal | AHD | |
---|---|---|---|---|---|---|---|---|---|---|---|---|---|---|---|
0 | 1 | 63 | 1 | typical | 145 | 233 | 1 | 2 | 150 | 0 | 2.3 | 3 | 0.0 | fixed | No |
1 | 2 | 67 | 1 | asymptomatic | 160 | 286 | 0 | 2 | 108 | 1 | 1.5 | 2 | 3.0 | normal | Yes |
2 | 3 | 67 | 1 | asymptomatic | 120 | 229 | 0 | 2 | 129 | 1 | 2.6 | 2 | 2.0 | reversable | Yes |
3 | 4 | 37 | 1 | nonanginal | 130 | 250 | 0 | 0 | 187 | 0 | 3.5 | 3 | 0.0 | normal | No |
4 | 5 | 41 | 0 | nontypical | 130 | 204 | 0 | 2 | 172 | 0 | 1.4 | 1 | 0.0 | normal | No |
5 | 6 | 56 | 1 | nontypical | 120 | 236 | 0 | 0 | 178 | 0 | 0.8 | 1 | 0.0 | normal | No |
6 | 7 | 62 | 0 | asymptomatic | 140 | 268 | 0 | 2 | 160 | 0 | 3.6 | 3 | 2.0 | normal | Yes |
7 | 8 | 57 | 0 | asymptomatic | 120 | 354 | 0 | 0 | 163 | 1 | 0.6 | 1 | 0.0 | normal | No |
8 | 9 | 63 | 1 | asymptomatic | 130 | 254 | 0 | 2 | 147 | 0 | 1.4 | 2 | 1.0 | reversable | Yes |
9 | 10 | 53 | 1 | asymptomatic | 140 | 203 | 1 | 2 | 155 | 1 | 3.1 | 3 | 0.0 | reversable | Yes |
df.tail(8)
Unnamed: 0 | Age | Sex | ChestPain | RestBP | Chol | Fbs | RestECG | MaxHR | ExAng | Oldpeak | Slope | Ca | Thal | AHD | |
---|---|---|---|---|---|---|---|---|---|---|---|---|---|---|---|
295 | 296 | 41 | 1 | nontypical | 120 | 157 | 0 | 0 | 182 | 0 | 0.0 | 1 | 0.0 | normal | No |
296 | 297 | 59 | 1 | asymptomatic | 164 | 176 | 1 | 2 | 90 | 0 | 1.0 | 2 | 2.0 | fixed | Yes |
297 | 298 | 57 | 0 | asymptomatic | 140 | 241 | 0 | 0 | 123 | 1 | 0.2 | 2 | 0.0 | reversable | Yes |
298 | 299 | 45 | 1 | typical | 110 | 264 | 0 | 0 | 132 | 0 | 1.2 | 2 | 0.0 | reversable | Yes |
299 | 300 | 68 | 1 | asymptomatic | 144 | 193 | 1 | 0 | 141 | 0 | 3.4 | 2 | 2.0 | reversable | Yes |
300 | 301 | 57 | 1 | asymptomatic | 130 | 131 | 0 | 0 | 115 | 1 | 1.2 | 2 | 1.0 | reversable | Yes |
301 | 302 | 57 | 0 | nontypical | 130 | 236 | 0 | 2 | 174 | 0 | 0.0 | 2 | 1.0 | normal | Yes |
302 | 303 | 38 | 1 | nonanginal | 138 | 175 | 0 | 0 | 173 | 0 | 0.0 | 1 | NaN | normal | No |
df.describe()
Unnamed: 0 | Age | Sex | RestBP | Chol | Fbs | RestECG | MaxHR | ExAng | Oldpeak | Slope | Ca | |
---|---|---|---|---|---|---|---|---|---|---|---|---|
count | 303.000000 | 303.000000 | 303.000000 | 303.000000 | 303.000000 | 303.000000 | 303.000000 | 303.000000 | 303.000000 | 303.000000 | 303.000000 | 299.000000 |
mean | 152.000000 | 54.438944 | 0.679868 | 131.689769 | 246.693069 | 0.148515 | 0.990099 | 149.607261 | 0.326733 | 1.039604 | 1.600660 | 0.672241 |
std | 87.612784 | 9.038662 | 0.467299 | 17.599748 | 51.776918 | 0.356198 | 0.994971 | 22.875003 | 0.469794 | 1.161075 | 0.616226 | 0.937438 |
min | 1.000000 | 29.000000 | 0.000000 | 94.000000 | 126.000000 | 0.000000 | 0.000000 | 71.000000 | 0.000000 | 0.000000 | 1.000000 | 0.000000 |
25% | 76.500000 | 48.000000 | 0.000000 | 120.000000 | 211.000000 | 0.000000 | 0.000000 | 133.500000 | 0.000000 | 0.000000 | 1.000000 | 0.000000 |
50% | 152.000000 | 56.000000 | 1.000000 | 130.000000 | 241.000000 | 0.000000 | 1.000000 | 153.000000 | 0.000000 | 0.800000 | 2.000000 | 0.000000 |
75% | 227.500000 | 61.000000 | 1.000000 | 140.000000 | 275.000000 | 0.000000 | 2.000000 | 166.000000 | 1.000000 | 1.600000 | 2.000000 | 1.000000 |
max | 303.000000 | 77.000000 | 1.000000 | 200.000000 | 564.000000 | 1.000000 | 2.000000 | 202.000000 | 1.000000 | 6.200000 | 3.000000 | 3.000000 |
df.index
RangeIndex(start=0, stop=303, step=1)
df.columns
Index(['Unnamed: 0', 'Age', 'Sex', 'ChestPain', 'RestBP', 'Chol', 'Fbs',
'RestECG', 'MaxHR', 'ExAng', 'Oldpeak', 'Slope', 'Ca', 'Thal', 'AHD'],
dtype='object')
# df.sort_index(axis=0, ascending=True)
# df.sort_index(axis=0, ascending=False)
df.sort_index(axis=1, ascending=True)
df.sort_index(axis=1, ascending=False)
Unnamed: 0 | Thal | Slope | Sex | RestECG | RestBP | Oldpeak | MaxHR | Fbs | ExAng | Chol | ChestPain | Ca | Age | AHD | |
---|---|---|---|---|---|---|---|---|---|---|---|---|---|---|---|
0 | 1 | fixed | 3 | 1 | 2 | 145 | 2.3 | 150 | 1 | 0 | 233 | typical | 0.0 | 63 | No |
1 | 2 | normal | 2 | 1 | 2 | 160 | 1.5 | 108 | 0 | 1 | 286 | asymptomatic | 3.0 | 67 | Yes |
2 | 3 | reversable | 2 | 1 | 2 | 120 | 2.6 | 129 | 0 | 1 | 229 | asymptomatic | 2.0 | 67 | Yes |
3 | 4 | normal | 3 | 1 | 0 | 130 | 3.5 | 187 | 0 | 0 | 250 | nonanginal | 0.0 | 37 | No |
4 | 5 | normal | 1 | 0 | 2 | 130 | 1.4 | 172 | 0 | 0 | 204 | nontypical | 0.0 | 41 | No |
... | ... | ... | ... | ... | ... | ... | ... | ... | ... | ... | ... | ... | ... | ... | ... |
298 | 299 | reversable | 2 | 1 | 0 | 110 | 1.2 | 132 | 0 | 0 | 264 | typical | 0.0 | 45 | Yes |
299 | 300 | reversable | 2 | 1 | 0 | 144 | 3.4 | 141 | 1 | 0 | 193 | asymptomatic | 2.0 | 68 | Yes |
300 | 301 | reversable | 2 | 1 | 0 | 130 | 1.2 | 115 | 0 | 1 | 131 | asymptomatic | 1.0 | 57 | Yes |
301 | 302 | normal | 2 | 0 | 2 | 130 | 0.0 | 174 | 0 | 0 | 236 | nontypical | 1.0 | 57 | Yes |
302 | 303 | normal | 1 | 1 | 0 | 138 | 0.0 | 173 | 0 | 0 | 175 | nonanginal | NaN | 38 | No |
303 rows × 15 columns
df.sort_values(by = 'Sex', ascending = False)
Unnamed: 0 | Age | Sex | ChestPain | RestBP | Chol | Fbs | RestECG | MaxHR | ExAng | Oldpeak | Slope | Ca | Thal | AHD | |
---|---|---|---|---|---|---|---|---|---|---|---|---|---|---|---|
15 | 16 | 57 | 1 | nonanginal | 150 | 168 | 0 | 0 | 174 | 0 | 1.6 | 1 | 0.0 | normal | No |
302 | 303 | 38 | 1 | nonanginal | 138 | 175 | 0 | 0 | 173 | 0 | 0.0 | 1 | NaN | normal | No |
0 | 1 | 63 | 1 | typical | 145 | 233 | 1 | 2 | 150 | 0 | 2.3 | 3 | 0.0 | fixed | No |
1 | 2 | 67 | 1 | asymptomatic | 160 | 286 | 0 | 2 | 108 | 1 | 1.5 | 2 | 3.0 | normal | Yes |
2 | 3 | 67 | 1 | asymptomatic | 120 | 229 | 0 | 2 | 129 | 1 | 2.6 | 2 | 2.0 | reversable | Yes |
... | ... | ... | ... | ... | ... | ... | ... | ... | ... | ... | ... | ... | ... | ... | ... |
291 | 292 | 55 | 0 | nontypical | 132 | 342 | 0 | 0 | 166 | 0 | 1.2 | 1 | 0.0 | normal | No |
18 | 19 | 48 | 0 | nonanginal | 130 | 275 | 0 | 0 | 139 | 0 | 0.2 | 1 | 0.0 | normal | No |
7 | 8 | 57 | 0 | asymptomatic | 120 | 354 | 0 | 0 | 163 | 1 | 0.6 | 1 | 0.0 | normal | No |
6 | 7 | 62 | 0 | asymptomatic | 140 | 268 | 0 | 2 | 160 | 0 | 3.6 | 3 | 2.0 | normal | Yes |
4 | 5 | 41 | 0 | nontypical | 130 | 204 | 0 | 2 | 172 | 0 | 1.4 | 1 | 0.0 | normal | No |
303 rows × 15 columns
df.T
0 | 1 | 2 | 3 | 4 | 5 | 6 | 7 | 8 | 9 | ... | 293 | 294 | 295 | 296 | 297 | 298 | 299 | 300 | 301 | 302 | |
---|---|---|---|---|---|---|---|---|---|---|---|---|---|---|---|---|---|---|---|---|---|
Unnamed: 0 | 1 | 2 | 3 | 4 | 5 | 6 | 7 | 8 | 9 | 10 | ... | 294 | 295 | 296 | 297 | 298 | 299 | 300 | 301 | 302 | 303 |
Age | 63 | 67 | 67 | 37 | 41 | 56 | 62 | 57 | 63 | 53 | ... | 63 | 63 | 41 | 59 | 57 | 45 | 68 | 57 | 57 | 38 |
Sex | 1 | 1 | 1 | 1 | 0 | 1 | 0 | 0 | 1 | 1 | ... | 1 | 0 | 1 | 1 | 0 | 1 | 1 | 1 | 0 | 1 |
ChestPain | typical | asymptomatic | asymptomatic | nonanginal | nontypical | nontypical | asymptomatic | asymptomatic | asymptomatic | asymptomatic | ... | asymptomatic | asymptomatic | nontypical | asymptomatic | asymptomatic | typical | asymptomatic | asymptomatic | nontypical | nonanginal |
RestBP | 145 | 160 | 120 | 130 | 130 | 120 | 140 | 120 | 130 | 140 | ... | 140 | 124 | 120 | 164 | 140 | 110 | 144 | 130 | 130 | 138 |
Chol | 233 | 286 | 229 | 250 | 204 | 236 | 268 | 354 | 254 | 203 | ... | 187 | 197 | 157 | 176 | 241 | 264 | 193 | 131 | 236 | 175 |
Fbs | 1 | 0 | 0 | 0 | 0 | 0 | 0 | 0 | 0 | 1 | ... | 0 | 0 | 0 | 1 | 0 | 0 | 1 | 0 | 0 | 0 |
RestECG | 2 | 2 | 2 | 0 | 2 | 0 | 2 | 0 | 2 | 2 | ... | 2 | 0 | 0 | 2 | 0 | 0 | 0 | 0 | 2 | 0 |
MaxHR | 150 | 108 | 129 | 187 | 172 | 178 | 160 | 163 | 147 | 155 | ... | 144 | 136 | 182 | 90 | 123 | 132 | 141 | 115 | 174 | 173 |
ExAng | 0 | 1 | 1 | 0 | 0 | 0 | 0 | 1 | 0 | 1 | ... | 1 | 1 | 0 | 0 | 1 | 0 | 0 | 1 | 0 | 0 |
Oldpeak | 2.3 | 1.5 | 2.6 | 3.5 | 1.4 | 0.8 | 3.6 | 0.6 | 1.4 | 3.1 | ... | 4.0 | 0.0 | 0.0 | 1.0 | 0.2 | 1.2 | 3.4 | 1.2 | 0.0 | 0.0 |
Slope | 3 | 2 | 2 | 3 | 1 | 1 | 3 | 1 | 2 | 3 | ... | 1 | 2 | 1 | 2 | 2 | 2 | 2 | 2 | 2 | 1 |
Ca | 0.0 | 3.0 | 2.0 | 0.0 | 0.0 | 0.0 | 2.0 | 0.0 | 1.0 | 0.0 | ... | 2.0 | 0.0 | 0.0 | 2.0 | 0.0 | 0.0 | 2.0 | 1.0 | 1.0 | NaN |
Thal | fixed | normal | reversable | normal | normal | normal | normal | normal | reversable | reversable | ... | reversable | normal | normal | fixed | reversable | reversable | reversable | reversable | normal | normal |
AHD | No | Yes | Yes | No | No | No | Yes | No | Yes | Yes | ... | Yes | Yes | No | Yes | Yes | Yes | Yes | Yes | Yes | No |
15 rows × 303 columns
df['Age']
0 63
1 67
2 67
3 37
4 41
..
298 45
299 68
300 57
301 57
302 38
Name: Age, Length: 303, dtype: int64
df[['Age', 'Sex']]
Age | Sex | |
---|---|---|
0 | 63 | 1 |
1 | 67 | 1 |
2 | 67 | 1 |
3 | 37 | 1 |
4 | 41 | 0 |
... | ... | ... |
298 | 45 | 1 |
299 | 68 | 1 |
300 | 57 | 1 |
301 | 57 | 0 |
302 | 38 | 1 |
303 rows × 2 columns
df[['Age', 'Sex']][0:2]
Age | Sex | |
---|---|---|
0 | 63 | 1 |
1 | 67 | 1 |
df[0:2][['Age']]
Age | |
---|---|
0 | 63 |
1 | 67 |
Data Selection#
df.loc[4]
# df
Unnamed: 0 5
Age 41
Sex 0
ChestPain nontypical
RestBP 130
Chol 204
Fbs 0
RestECG 2
MaxHR 172
ExAng 0
Oldpeak 1.4
Slope 1
Ca 0.0
Thal normal
AHD No
Name: 4, dtype: object
df.loc[[3,5], ['Age', 'Fbs']]
Age | Fbs | |
---|---|---|
3 | 37 | 0 |
5 | 56 | 0 |
df.iloc[5]
Unnamed: 0 6
Age 56
Sex 1
ChestPain nontypical
RestBP 120
Chol 236
Fbs 0
RestECG 0
MaxHR 178
ExAng 0
Oldpeak 0.8
Slope 1
Ca 0.0
Thal normal
AHD No
Name: 5, dtype: object
df.iloc[5, 3]
'nontypical'
# df.iloc[5:7, 3:7]
# df.iloc[[5, 7, 9], 3:7]
# df.iloc[:, 3]
df.iloc[3: 5, :]
# df.loc[5:7, 3:7]
Unnamed: 0 | Age | Sex | ChestPain | RestBP | Chol | Fbs | RestECG | MaxHR | ExAng | Oldpeak | Slope | Ca | Thal | AHD | |
---|---|---|---|---|---|---|---|---|---|---|---|---|---|---|---|
3 | 4 | 37 | 1 | nonanginal | 130 | 250 | 0 | 0 | 187 | 0 | 3.5 | 3 | 0.0 | normal | No |
4 | 5 | 41 | 0 | nontypical | 130 | 204 | 0 | 2 | 172 | 0 | 1.4 | 1 | 0.0 | normal | No |
df.iloc[1, 1]
df.at[1, 'Age'] # Get scalar values. It's a very fast loc
df.iat[1, 1] # Get scalar values. It's a very fast iloc
np.int64(67)
df['Age'] > 50
0 True
1 True
2 True
3 False
4 False
...
298 False
299 True
300 True
301 True
302 False
Name: Age, Length: 303, dtype: bool
df[df.Age > 50]
Unnamed: 0 | Age | Sex | ChestPain | RestBP | Chol | Fbs | RestECG | MaxHR | ExAng | Oldpeak | Slope | Ca | Thal | AHD | |
---|---|---|---|---|---|---|---|---|---|---|---|---|---|---|---|
0 | 1 | 63 | 1 | typical | 145 | 233 | 1 | 2 | 150 | 0 | 2.3 | 3 | 0.0 | fixed | No |
1 | 2 | 67 | 1 | asymptomatic | 160 | 286 | 0 | 2 | 108 | 1 | 1.5 | 2 | 3.0 | normal | Yes |
2 | 3 | 67 | 1 | asymptomatic | 120 | 229 | 0 | 2 | 129 | 1 | 2.6 | 2 | 2.0 | reversable | Yes |
5 | 6 | 56 | 1 | nontypical | 120 | 236 | 0 | 0 | 178 | 0 | 0.8 | 1 | 0.0 | normal | No |
6 | 7 | 62 | 0 | asymptomatic | 140 | 268 | 0 | 2 | 160 | 0 | 3.6 | 3 | 2.0 | normal | Yes |
... | ... | ... | ... | ... | ... | ... | ... | ... | ... | ... | ... | ... | ... | ... | ... |
296 | 297 | 59 | 1 | asymptomatic | 164 | 176 | 1 | 2 | 90 | 0 | 1.0 | 2 | 2.0 | fixed | Yes |
297 | 298 | 57 | 0 | asymptomatic | 140 | 241 | 0 | 0 | 123 | 1 | 0.2 | 2 | 0.0 | reversable | Yes |
299 | 300 | 68 | 1 | asymptomatic | 144 | 193 | 1 | 0 | 141 | 0 | 3.4 | 2 | 2.0 | reversable | Yes |
300 | 301 | 57 | 1 | asymptomatic | 130 | 131 | 0 | 0 | 115 | 1 | 1.2 | 2 | 1.0 | reversable | Yes |
301 | 302 | 57 | 0 | nontypical | 130 | 236 | 0 | 2 | 174 | 0 | 0.0 | 2 | 1.0 | normal | Yes |
209 rows × 15 columns
df2 = df.copy()
df2 = df2.iloc[0: 5, :]
df2
Unnamed: 0 | Age | Sex | ChestPain | RestBP | Chol | Fbs | RestECG | MaxHR | ExAng | Oldpeak | Slope | Ca | Thal | AHD | |
---|---|---|---|---|---|---|---|---|---|---|---|---|---|---|---|
0 | 1 | 63 | 1 | typical | 145 | 233 | 1 | 2 | 150 | 0 | 2.3 | 3 | 0.0 | fixed | No |
1 | 2 | 67 | 1 | asymptomatic | 160 | 286 | 0 | 2 | 108 | 1 | 1.5 | 2 | 3.0 | normal | Yes |
2 | 3 | 67 | 1 | asymptomatic | 120 | 229 | 0 | 2 | 129 | 1 | 2.6 | 2 | 2.0 | reversable | Yes |
3 | 4 | 37 | 1 | nonanginal | 130 | 250 | 0 | 0 | 187 | 0 | 3.5 | 3 | 0.0 | normal | No |
4 | 5 | 41 | 0 | nontypical | 130 | 204 | 0 | 2 | 172 | 0 | 1.4 | 1 | 0.0 | normal | No |
# dfa['A'] = list(range(len(dfa.index))) # use this form to create a new column
df2['cp'] = ['three', 'two', 'one', 'one', 'zero']
df2
df2.cp.isin(['one', 'zero'])
0 False
1 False
2 True
3 True
4 True
Name: cp, dtype: bool
df2[df2.cp.isin(['one', 'zero'])]
Unnamed: 0 | Age | Sex | ChestPain | RestBP | Chol | Fbs | RestECG | MaxHR | ExAng | Oldpeak | Slope | Ca | Thal | AHD | cp | |
---|---|---|---|---|---|---|---|---|---|---|---|---|---|---|---|---|
2 | 3 | 67 | 1 | asymptomatic | 120 | 229 | 0 | 2 | 129 | 1 | 2.6 | 2 | 2.0 | reversable | Yes | one |
3 | 4 | 37 | 1 | nonanginal | 130 | 250 | 0 | 0 | 187 | 0 | 3.5 | 3 | 0.0 | normal | No | one |
4 | 5 | 41 | 0 | nontypical | 130 | 204 | 0 | 2 | 172 | 0 | 1.4 | 1 | 0.0 | normal | No | zero |
Missing Data#
df1 = df.reindex(index=range(0, 4), columns=list(df.columns) + ['E'])
df1.loc[2:3, 'E'] = 1
df1
Unnamed: 0 | Age | Sex | ChestPain | RestBP | Chol | Fbs | RestECG | MaxHR | ExAng | Oldpeak | Slope | Ca | Thal | AHD | E | |
---|---|---|---|---|---|---|---|---|---|---|---|---|---|---|---|---|
0 | 1 | 63 | 1 | typical | 145 | 233 | 1 | 2 | 150 | 0 | 2.3 | 3 | 0.0 | fixed | No | NaN |
1 | 2 | 67 | 1 | asymptomatic | 160 | 286 | 0 | 2 | 108 | 1 | 1.5 | 2 | 3.0 | normal | Yes | NaN |
2 | 3 | 67 | 1 | asymptomatic | 120 | 229 | 0 | 2 | 129 | 1 | 2.6 | 2 | 2.0 | reversable | Yes | 1.0 |
3 | 4 | 37 | 1 | nonanginal | 130 | 250 | 0 | 0 | 187 | 0 | 3.5 | 3 | 0.0 | normal | No | 1.0 |
df1.dropna(how='any')
Unnamed: 0 | Age | Sex | ChestPain | RestBP | Chol | Fbs | RestECG | MaxHR | ExAng | Oldpeak | Slope | Ca | Thal | AHD | E | |
---|---|---|---|---|---|---|---|---|---|---|---|---|---|---|---|---|
2 | 3 | 67 | 1 | asymptomatic | 120 | 229 | 0 | 2 | 129 | 1 | 2.6 | 2 | 2.0 | reversable | Yes | 1.0 |
3 | 4 | 37 | 1 | nonanginal | 130 | 250 | 0 | 0 | 187 | 0 | 3.5 | 3 | 0.0 | normal | No | 1.0 |
pd.isna(df1)
Unnamed: 0 | Age | Sex | ChestPain | RestBP | Chol | Fbs | RestECG | MaxHR | ExAng | Oldpeak | Slope | Ca | Thal | AHD | E | |
---|---|---|---|---|---|---|---|---|---|---|---|---|---|---|---|---|
0 | False | False | False | False | False | False | False | False | False | False | False | False | False | False | False | True |
1 | False | False | False | False | False | False | False | False | False | False | False | False | False | False | False | True |
2 | False | False | False | False | False | False | False | False | False | False | False | False | False | False | False | False |
3 | False | False | False | False | False | False | False | False | False | False | False | False | False | False | False | False |
df1.fillna(value=2)
Unnamed: 0 | Age | Sex | ChestPain | RestBP | Chol | Fbs | RestECG | MaxHR | ExAng | Oldpeak | Slope | Ca | Thal | AHD | E | |
---|---|---|---|---|---|---|---|---|---|---|---|---|---|---|---|---|
0 | 1 | 63 | 1 | typical | 145 | 233 | 1 | 2 | 150 | 0 | 2.3 | 3 | 0.0 | fixed | No | 2.0 |
1 | 2 | 67 | 1 | asymptomatic | 160 | 286 | 0 | 2 | 108 | 1 | 1.5 | 2 | 3.0 | normal | Yes | 2.0 |
2 | 3 | 67 | 1 | asymptomatic | 120 | 229 | 0 | 2 | 129 | 1 | 2.6 | 2 | 2.0 | reversable | Yes | 1.0 |
3 | 4 | 37 | 1 | nonanginal | 130 | 250 | 0 | 0 | 187 | 0 | 3.5 | 3 | 0.0 | normal | No | 1.0 |
Operations#
df1.mean(numeric_only=True)
Unnamed: 0 2.500
Age 58.500
Sex 1.000
RestBP 138.750
Chol 249.500
Fbs 0.250
RestECG 1.500
MaxHR 143.500
ExAng 0.500
Oldpeak 2.475
Slope 2.500
Ca 1.250
E 1.000
dtype: float64
df1.mean(axis = 1, numeric_only=True)
0 50.108333
1 52.791667
2 43.046154
3 47.423077
dtype: float64
# df1.sub([10 for _ in range(4)], axis = 'index')
df1.mul([10 for _ in range(4)], axis = 'index')
Unnamed: 0 | Age | Sex | ChestPain | RestBP | Chol | Fbs | RestECG | MaxHR | ExAng | Oldpeak | Slope | Ca | Thal | AHD | E | |
---|---|---|---|---|---|---|---|---|---|---|---|---|---|---|---|---|
0 | 10 | 630 | 10 | typicaltypicaltypicaltypicaltypicaltypicaltypi... | 1450 | 2330 | 10 | 20 | 1500 | 0 | 23.0 | 30 | 0.0 | fixedfixedfixedfixedfixedfixedfixedfixedfixedf... | NoNoNoNoNoNoNoNoNoNo | NaN |
1 | 20 | 670 | 10 | asymptomaticasymptomaticasymptomaticasymptomat... | 1600 | 2860 | 0 | 20 | 1080 | 10 | 15.0 | 20 | 30.0 | normalnormalnormalnormalnormalnormalnormalnorm... | YesYesYesYesYesYesYesYesYesYes | NaN |
2 | 30 | 670 | 10 | asymptomaticasymptomaticasymptomaticasymptomat... | 1200 | 2290 | 0 | 20 | 1290 | 10 | 26.0 | 20 | 20.0 | reversablereversablereversablereversablerevers... | YesYesYesYesYesYesYesYesYesYes | 10.0 |
3 | 40 | 370 | 10 | nonanginalnonanginalnonanginalnonanginalnonang... | 1300 | 2500 | 0 | 0 | 1870 | 0 | 35.0 | 30 | 0.0 | normalnormalnormalnormalnormalnormalnormalnorm... | NoNoNoNoNoNoNoNoNoNo | 10.0 |
df1
Unnamed: 0 | Age | Sex | ChestPain | RestBP | Chol | Fbs | RestECG | MaxHR | ExAng | Oldpeak | Slope | Ca | Thal | AHD | E | |
---|---|---|---|---|---|---|---|---|---|---|---|---|---|---|---|---|
0 | 1 | 63 | 1 | typical | 145 | 233 | 1 | 2 | 150 | 0 | 2.3 | 3 | 0.0 | fixed | No | NaN |
1 | 2 | 67 | 1 | asymptomatic | 160 | 286 | 0 | 2 | 108 | 1 | 1.5 | 2 | 3.0 | normal | Yes | NaN |
2 | 3 | 67 | 1 | asymptomatic | 120 | 229 | 0 | 2 | 129 | 1 | 2.6 | 2 | 2.0 | reversable | Yes | 1.0 |
3 | 4 | 37 | 1 | nonanginal | 130 | 250 | 0 | 0 | 187 | 0 | 3.5 | 3 | 0.0 | normal | No | 1.0 |
df1.apply(np.cumsum)
Unnamed: 0 | Age | Sex | ChestPain | RestBP | Chol | Fbs | RestECG | MaxHR | ExAng | Oldpeak | Slope | Ca | Thal | AHD | E | |
---|---|---|---|---|---|---|---|---|---|---|---|---|---|---|---|---|
0 | 1 | 63 | 1 | typical | 145 | 233 | 1 | 2 | 150 | 0 | 2.3 | 3 | 0.0 | fixed | No | NaN |
1 | 3 | 130 | 2 | typicalasymptomatic | 305 | 519 | 1 | 4 | 258 | 1 | 3.8 | 5 | 3.0 | fixednormal | NoYes | NaN |
2 | 6 | 197 | 3 | typicalasymptomaticasymptomatic | 425 | 748 | 1 | 6 | 387 | 2 | 6.4 | 7 | 5.0 | fixednormalreversable | NoYesYes | 1.0 |
3 | 10 | 234 | 4 | typicalasymptomaticasymptomaticnonanginal | 555 | 998 | 1 | 6 | 574 | 2 | 9.9 | 10 | 5.0 | fixednormalreversablenormal | NoYesYesNo | 2.0 |
df1.Age.apply(lambda x: x*365.25)
0 23010.75
1 24471.75
2 24471.75
3 13514.25
Name: Age, dtype: float64
s = pd.Series(np.random.randint(0, 7, size=10))
s
0 6
1 6
2 6
3 3
4 5
5 2
6 4
7 0
8 6
9 3
dtype: int64
s.value_counts()
6 4
3 2
5 1
2 1
4 1
0 1
Name: count, dtype: int64
s = pd.Series(['A', 'B', 'C', 'Aaba', 'Baca', np.nan, 'CABA', 'dog', 'cat'])
s
0 A
1 B
2 C
3 Aaba
4 Baca
5 NaN
6 CABA
7 dog
8 cat
dtype: object
s.str.lower()
0 a
1 b
2 c
3 aaba
4 baca
5 NaN
6 caba
7 dog
8 cat
dtype: object
Merge#
df2 = pd.DataFrame(np.random.randn(10, 4))
pieces = [df2[:3], df2[3:7], df2[7:]]
pieces
[ 0 1 2 3
0 1.297844 0.361677 1.517966 -0.520389
1 1.482297 1.573645 -1.042870 -0.467312
2 0.534757 -0.035592 1.614892 0.840001,
0 1 2 3
3 -0.834683 0.207694 -1.587102 -0.203180
4 -0.470024 -1.150068 0.061028 -0.807551
5 -0.561795 -0.724152 -2.046658 -1.955907
6 0.716297 0.022458 -1.321205 -1.268533,
0 1 2 3
7 0.272238 -0.806136 -2.503119 -0.788643
8 1.461160 -1.577110 0.738925 1.031984
9 0.487780 0.098689 0.903801 -0.424845]
pd.concat([pieces[2], pieces[1], pieces[0]])
0 | 1 | 2 | 3 | |
---|---|---|---|---|
7 | 0.272238 | -0.806136 | -2.503119 | -0.788643 |
8 | 1.461160 | -1.577110 | 0.738925 | 1.031984 |
9 | 0.487780 | 0.098689 | 0.903801 | -0.424845 |
3 | -0.834683 | 0.207694 | -1.587102 | -0.203180 |
4 | -0.470024 | -1.150068 | 0.061028 | -0.807551 |
5 | -0.561795 | -0.724152 | -2.046658 | -1.955907 |
6 | 0.716297 | 0.022458 | -1.321205 | -1.268533 |
0 | 1.297844 | 0.361677 | 1.517966 | -0.520389 |
1 | 1.482297 | 1.573645 | -1.042870 | -0.467312 |
2 | 0.534757 | -0.035592 | 1.614892 | 0.840001 |
left = pd.DataFrame({'key': ['foo', 'foo'], 'lval': [1, 2]})
right = pd.DataFrame({'key': ['foo', 'foo'], 'rval': [4, 5]})
left
key | lval | |
---|---|---|
0 | foo | 1 |
1 | foo | 2 |
right
key | rval | |
---|---|---|
0 | foo | 4 |
1 | foo | 5 |
pd.merge(left, right, on='key')
key | lval | rval | |
---|---|---|---|
0 | foo | 1 | 4 |
1 | foo | 1 | 5 |
2 | foo | 2 | 4 |
3 | foo | 2 | 5 |
left = pd.DataFrame({'key': ['foo', 'bar'], 'lval': [1, 2]})
right = pd.DataFrame({'key': ['foo', 'bar'], 'rval': [4, 5]})
pd.merge(left, right, on='key')
key | lval | rval | |
---|---|---|---|
0 | foo | 1 | 4 |
1 | bar | 2 | 5 |
left
key | lval | |
---|---|---|
0 | foo | 1 |
1 | bar | 2 |
right
key | rval | |
---|---|---|
0 | foo | 4 |
1 | bar | 5 |
df = pd.DataFrame(np.random.randn(8, 4), columns=['A', 'B', 'C', 'D'])
df
A | B | C | D | |
---|---|---|---|---|
0 | -0.045089 | 0.958249 | 0.635655 | 0.302620 |
1 | -1.309998 | -0.574274 | -0.022655 | 1.040313 |
2 | 0.049250 | 0.386385 | 0.241335 | -0.506924 |
3 | -1.019096 | -0.067548 | 0.609384 | -0.410076 |
4 | -0.447381 | 1.461726 | 1.934281 | 0.122305 |
5 | -0.058097 | 0.412266 | 1.322237 | -0.993059 |
6 | 1.463590 | 1.054467 | -0.076414 | 0.037145 |
7 | 1.195363 | -0.402542 | 1.214808 | -0.380158 |
Grouping#
df = pd.DataFrame({'A': ['foo', 'bar', 'foo', 'bar',
'foo', 'bar', 'foo', 'foo'],
'B': ['one', 'one', 'two', 'three',
'two', 'two', 'one', 'three'],
'C': np.random.randn(8),
'D': np.random.randn(8)})
df
A | B | C | D | |
---|---|---|---|---|
0 | foo | one | 1.050997 | -1.837274 |
1 | bar | one | -1.701427 | -1.538114 |
2 | foo | two | -1.011101 | 0.981054 |
3 | bar | three | -1.244928 | -1.236973 |
4 | foo | two | -1.037760 | -1.314032 |
5 | bar | two | 1.746614 | 0.117936 |
6 | foo | one | -0.685186 | -1.459810 |
7 | foo | three | -0.854793 | 0.935833 |
df.groupby('A').sum()
B | C | D | |
---|---|---|---|
A | |||
bar | onethreetwo | -1.199740 | -2.657152 |
foo | onetwotwoonethree | -2.537843 | -2.694229 |
df.groupby(['A', 'B']).sum()
C | D | ||
---|---|---|---|
A | B | ||
bar | one | -1.701427 | -1.538114 |
three | -1.244928 | -1.236973 | |
two | 1.746614 | 0.117936 | |
foo | one | 0.365811 | -3.297084 |
three | -0.854793 | 0.935833 | |
two | -2.048860 | -0.332978 |
Plotting#
df.groupby('A').sum()['C'].plot(kind='bar')
<Axes: xlabel='A'>
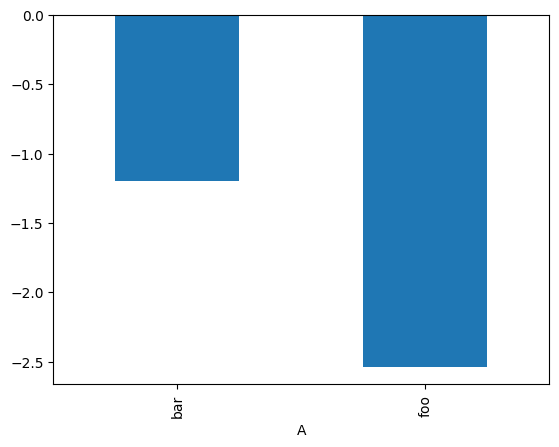
df2 = pd.DataFrame(np.random.rand(10, 4), columns=['a', 'b', 'c', 'd'])
df2.plot.bar()
<Axes: >
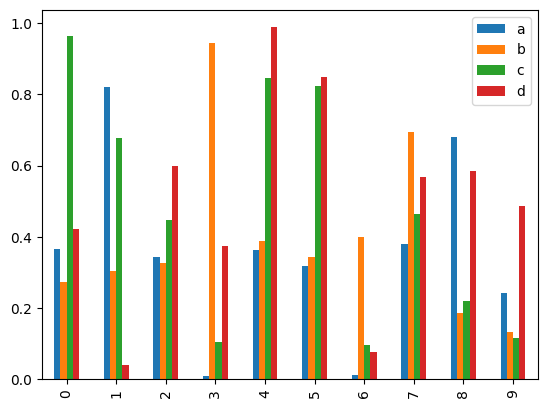
df2.plot.bar(stacked=True);
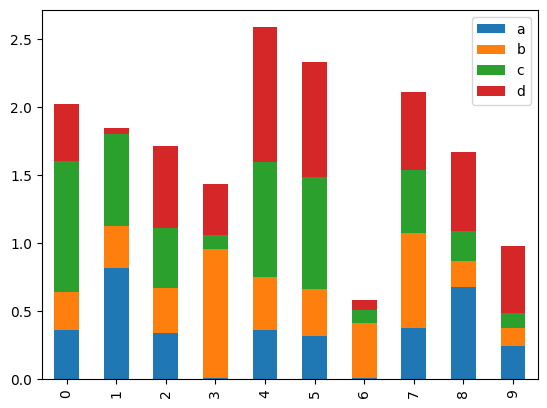
df2.plot.barh(stacked=True)
<Axes: >
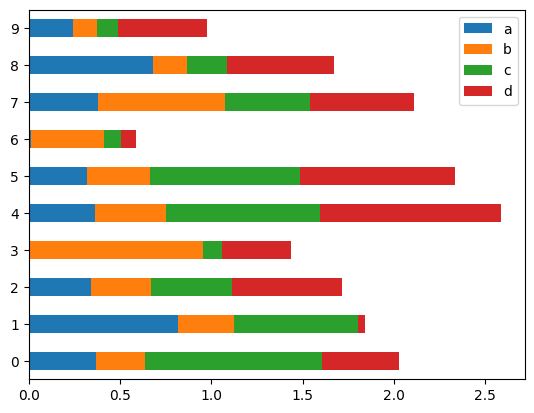
File I/O#
df = pd.read_csv('heart.csv')
df.head()
Unnamed: 0 | Age | Sex | ChestPain | RestBP | Chol | Fbs | RestECG | MaxHR | ExAng | Oldpeak | Slope | Ca | Thal | AHD | |
---|---|---|---|---|---|---|---|---|---|---|---|---|---|---|---|
0 | 1 | 63 | 1 | typical | 145 | 233 | 1 | 2 | 150 | 0 | 2.3 | 3 | 0.0 | fixed | No |
1 | 2 | 67 | 1 | asymptomatic | 160 | 286 | 0 | 2 | 108 | 1 | 1.5 | 2 | 3.0 | normal | Yes |
2 | 3 | 67 | 1 | asymptomatic | 120 | 229 | 0 | 2 | 129 | 1 | 2.6 | 2 | 2.0 | reversable | Yes |
3 | 4 | 37 | 1 | nonanginal | 130 | 250 | 0 | 0 | 187 | 0 | 3.5 | 3 | 0.0 | normal | No |
4 | 5 | 41 | 0 | nontypical | 130 | 204 | 0 | 2 | 172 | 0 | 1.4 | 1 | 0.0 | normal | No |
# df.to_csv('heart_copy.csv', index = False)
# df1 = df[:10]
# df2 = df[10:20]
# df3 = df[20:40]
# with pd.ExcelWriter('path_to_file.xlsx') as writer:
# df1.to_excel(writer, sheet_name='Sheet1', index = False)
# df2.to_excel(writer, sheet_name='Sheet2', index = False)
# df3.to_excel(writer, sheet_name='Sheet3')